As the world continues to fight to contain the spread of COVID-19, our team at the Boulevard Consulting Group has, like millions of others, been working remotely since mid-March and staying at home in an effort to practice social distancing. As might have been the case for you, more time at home has meant plenty of time to binge-watch. Although we tend to watch shows as an escape from current events and responsibilities - quite successfully with Netflix's Tiger King - we couldn't help but find provoking parallels between our current world and the science-fiction realities portrayed in some of our favorite new television series and classic movies. Though the fictional universes aren't identical to the data-driven reality of today, it's helpful to reflect on the similarities and differences, and to ponder how best we can navigate today's challenges through this lens.
Minority Report and Predictive Policing
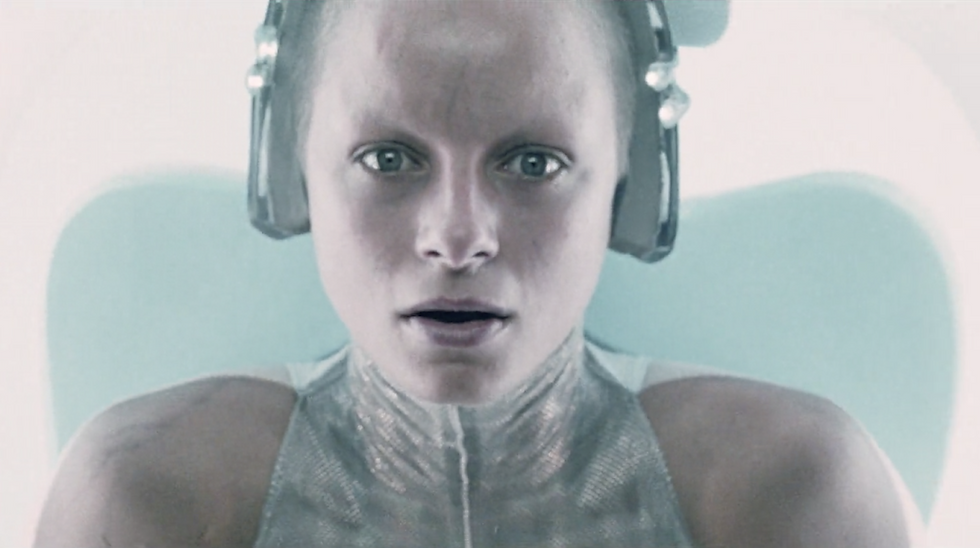
Agatha, one of the precogs in Minority Report (2002)
Minority Report, the acclaimed 2002 film directed by Steven Spielberg and starring Tom Cruise, is oft-cited as an example of the use of big data and artificial intelligence to construct a data-driven surveillance state. Set here in Washington, DC in 2054, Minority Report centers around the PreCrime police department, headed by Tom Cruise's character, John Anderton. The PreCrime division utilizes the visions of a trio of psychics called "precogs" to identify and stop criminal activity before it occurs. Although the manner in which the crimes are predicted is odd - visions from orphans with psychic powers spending their existence suspended in a photon milk bath - the idea is not dissimilar to machine learning models in development today.
Predictive policing programs have been explored by the LAPD, NYPD, and Chicago Police Department, among others. But, such efforts have been widely-criticized for a lack of transparency, questionable legality (issues of probable cause under the 4th Amendment), and clouded by bias. While Minority Report largely skirts the data and modeling issues by encapsulating its black box model with its "precogs," the movie acknowledges the ethical issues of free will vs. determinism by punishing people for crimes that they didn't yet commit. Real-world implementations of predictive policing raises not only this philosophical issue, but often malfunctions due to inadequate or biased data inputs and amplifies racially-biased policing practices historically in-place. PredPol, one of the firms pioneering predictive policing software and contracting with the LAPD and hundreds of other police departments, claims to solve privacy and civil rights issues by limiting the types of data used:
PredPol uses ONLY THREE data points - crime type, crime location, and crime date/time - to create its predictions. No personally identifiable information is ever used. No demographic, ethnic or socio-economic information is ever used. This eliminates the possibility for privacy or civil rights violations seen with other intelligence-led or predictive policing models.
Though explicit demographic data is not leaked to the model, this approach does nothing to solve pre-existing implicit biases. For example, let's consider the variable crime location. Crime location at first may seem to be an unbiased data point, but due to de facto segregation, location or neighborhood data is often a strong proxy for racial attributes. Furthermore, predictive policing models often suffer from data collection biases; if police departments historically target certain populations at a higher rate with policies such as Stop and Frisk or broken window tactics, the algorithms can amplify those patterns with its positive feedback loop.
As outlined in initial research from PredPol Chief Data Scientist, George Mohler et al., the clustering algorithms adopted for use in predictive policing models have traditionally been used by seismologists to predict earthquake aftershocks. Once again, the challenge lies within the quality of the data itself. For examining earthquakes, real-time seismic activity data recorded by the US Geological Survey covers the whole country; if an earthquake occurs it's recorded. Crime statistics, unfortunately, aren't as uniformly captured. Whether and how a crime is recorded can depend on a number of subjective factors: how likely are members of the community to report crimes to the authorities? what is the density of police presence in that community? are authorities employing broken window tactics that crack down on petty crime that may otherwise go unrecorded? Thus, once again, the algorithms and the model reinforce the data and any issues therein.
Context Matters
So what can be done to mitigate these issues of ethics, consistency, and legality? Cathy O'Neil, data science consultant and author of Weapons of Math Destruction, proposes an ethical matrix for helping organizations think about and deal with these issues. As O'Neil explains, "algorithms themselves don't have ethics [poor or otherwise] but algorithms used in a context by humans do have ethics." When PredPol cites its non-use of demographic data as evidence of its lack of bias, the company is showcasing the model's results without full acknowledgement of its context. Regardless of the model, there are always going to be some tradeoff; do you want to minimize false positives or false negatives? do you want the highest possible accuracy? In Minority Report, the film's title comes from the situation in which one of the three precogs sees a different vision than the other two. This "minority report" is overruled by the visions of the other two precogs and the system reports the majority prediction to the PreCrime authorities to act upon. What if in fact the minority report is the correct one?
In the movie, an innocent person is then doomed to spend his/her eternity in a virtual reality prison. As we've seen far too often, the mistakes in our world can be just as costly.
While predictive modeling can be an extremely powerful and useful tool, "with great power comes great responsibility."
When developing and utilizing a data science tool, remember to consider the following factors:
Are we complying with laws and regulations, including individuals' privacy rights?
Is data aggregation and linkage creating privacy or data security classification issues?
Are there any identifiable biases in the way the data is collected or used in the model? Can these issues be minimized?
How transparent is the model?
How are the model's predictions going to be utilized?
What are the consequences of such results and the associated cost-benefits?
Machine learning and artificial intelligence systems certainly have a multitude of applications as people seek to use the growing amount of data collected in the world today. However, these tools are not one size fits all. Context matters and it's important to start with a clear understanding of the opportunities and risks throughout your project from data collection to model development to end-use.
We at the Boulevard Consulting Group pride ourselves on providing not only the technical expertise to utilize the latest in machine learning techniques, but also the strategy and operations experience to craft solutions customized and optimized for each client. If you're interested in learning more about Boulevard's services, or simply want to talk about data ethics in sci-fi movies, visit our website and reach out!
About the Author
Will Karnasiewicz is an Associate at Boulevard with a focus on data science, software engineering, and financial valuation. He has earned a M.S. in Finance from Washington University in St. Louis, and a B.A. in Economics from Georgetown University. He is a holder of the chartered financial analyst (CFA®) designation from the CFA® Institute.
Commentaires